In this article, titled “Statistics II Solved Paper 2014 FBISE,” we will walk through a comprehensive analysis of the solved paper, addressing various aspects of statistics as encountered in the 2013 FBISE exam. The post will cover multiple-choice questions (MCQs), short-answer questions, numerical problems, and extensive questions on fundamental topics such as probability distribution, binomial and hypergeometric distributions, normal distribution, sampling distribution, inferential statistics (hypothesis testing and estimation), and association using the Chi-square test.
Each topic will be explained in detail, with solutions to guide students through the process of answering such questions efficiently. Whether you’re preparing for an exam or just brushing up on your statistics knowledge, this post serves as a helpful resource for mastering key statistical concepts and methodologies. This topic is equally important for the students of statistics across all the major Boards and Universities such as FBISE, BISERWP, BISELHR, MU, DU, PU, NCERT, CBSE & others & across all the statistics, business & finance disciplines.
Table of Contents
Statistics II Solved Paper 2014 FBISE
MCQS
Fill the relevant bubble against each question according to curriculum: | |||
1 | When a coin and a die are rolled together. all possible outcomes are: | ||
A) | 2 | B) | 6 |
C) | 8 | D) | 12 |
2 | If P(A/B)=P(A) and P(B/A)=P(B), then A and B are: | ||
A) | Mutually exclusive events | B) | Equally likely events |
C) | Independent events | D) | Dependent events |
3 | If P(B/A)=0.25 and P( A∩B) = 0.20, then P(A) is: | ||
A) | 0.05 | B) | 0.80 |
C) | 0.95 | D) | 0.75 |
4 | Which of the following cannot be taken as probability of an event? | ||
A) | 0 | B) | 0.5 |
C) | 1 | D) | -0.5 |
5 | Given E(X)=5 and E(Y)= 2, then E(X-Y) iS: | ||
A) | 3 | B) | 7 |
C) | 5 | D) | -2 |
6 | If “c” Is a constant In a continuous probability distribution p(x = C) is: | ||
A) | Zero | B) | Positive |
C) | One | D) | Impossible |
7 | What Is the value of Var (8 – 4X)? | ||
A) | 8+4 Var(X) | B) | 8+16 Var (X) |
C) | 16 Var (X) | D) | None of these |
8 | For a binomial probability distribution with n=5,p=1/2, then P(X=3.5) is: | ||
A) | 0.4593 | B) | 0 |
C) | 0.1986 | D) | 1.0831 |
9 | If X Is hypergeometric random variable with N=20, K=4 and n=6, it can assume any discrete value from: | ||
A) | 0,1,2,….6 | B) | 0,1,2,3,4 |
C) | 1,2,3,4 | D) | 1,2,3,…6 |
10 | The points of inflection of the normal curve with mean μ and variance σ² lie at: | ||
A) | μ- σ and μ+ σ | B) | μ- 2σ and μ+ 2σ |
C) | μ- 3σ and μ+ 3σ | D) | None of these |
11 | If a normal distribution with μ = 200 has P(X>225)=0.1587, then P(X<175) equal to: | ||
A) | 0.3413 | B) | 0.8413 |
C) | 0.1587 | D) | 0.5000 |
12 | A border patrol check point that stops every passenger van is using: | ||
A) | Simple Random sampling | B) | Systematic sampling |
C) | Stratified sampling | D) | Complete enumeration |
13 | The difference between the values of a statistic and a parameter is called: | ||
A) | Sampling error | B) | Non-sampling error |
C) | Standard error | D) | Bias |
14 | The level of confidence is denoted by: | ||
A) | α | B) | 1 – α |
C) | β | D) | 1 – β |
15 | A failing student is passed by an examiner. It is an example of: | ||
A) | Type I error | B) | Type II error |
C) | Correct decision | D) | Sampling error |
16 | In an unpaired sample t-test with sample sizes n1 = 11 and n2 = 11, the value of tabulated t should be obtained for: | ||
A) | 10 degree of freedom | B) | 21 degree of freedom |
C) | 22 degree of freedom | D) | 20 degree of freedom |
17 | For a 3 x 3 contingency table, the number of cells in the table is: | ||
A) | 3 | B) | 6 |
C) | 9 | D) | 4 |
Short Questions
(i) A company has 8 directors. In how many ways can the following be selected:
a. Chairman, MD, Executive Director
b. Three members to form a sub-committee
Solution: (a)
Data: n = 8 , r = 3
- Case of Permutation
There are 336 ways that t selects a Chairman, MD, Executive Director
- Case of Combination
So, there are 56 ways to select three members for the sub-committee.
(ii) Define the following:
a. Sample space
b. Mutually Exclusive events
c. Independent events
Answer:
Sample Space
All possible outcomes of a random experiment are called sample space. It is denoted by η(S). For example, in case of tossing a coin, the sample space will be 2 and in case of throwing a die, the sample space will be 6.
Mutually Exclusive Events
If two events A and B have nothing common between them, then the events are called mutually exclusive events or we can say that both event cannot happen at the same time. For example, in tossing a coin, head and tail both cannot be appear at the same time. In statistical notation, we can notate it as:
Independent Event
Independent event is an event that is not dependent to other event or we can say that changes in other event does not change the occurrence of independent event.
(iii) Consider two events A and B such that P(A̅)=1/3, P(B/A) =3/5 and P (B/ A̅) =4/5. Find P(B)
Solution:
Data
P(A̅)=1/3, P(A) = 2/3, P(B/A) =3/5 and P (B/ A̅) =4/5, P(B) =?
Formula
Substitute the values
(iv) The probability that a boy will pass an examination is 3/5 and that for a girl is 2/5. What is the probability that at least one of them passes the examination?
Solution:
Alternative Method
(v) What is a Probability Distribution? In how many ways can you express a probability distribution?
Answer:
Probability Distribution
Probability distribution explains the probability or chance of occurrence of each value of the random variable or we can say that values of the random variable and their corresponding probabilities are presented in probability distribution. Probability distribution can be presented in following ways:
Probability Distribution for Discrete Random Variable/Probability Mass Function PMF
Probability distribution which explains the discrete random variable which has countable values and their corresponding probabilities also called probability mass function. It calculates the specific value of X.
Probability Distribution for Continuous Random Variable/Probability Density Function PDF
Probability distribution which explains the continuous random variable which has measurable values and their corresponding probabilities also called probability density function. It calculates the value within range (a and b)
Probability Distribution Function for Discrete and Continuous Random Variable/Cumulative Distribution Function CDF
Probability distribution which explains both discrete and continuous random variable which has countable and measurable values and their corresponding probabilities also called probability cumulative distribution function.
(vi) Let X has the following probability distribution:
x | -1 | 0 | 1 | 2 | 3 |
f(x) | 0.125 | 0.50 | 0.20 | 0.05 | 0.125 |
Find the probability distribution of:
a. (2x-3)
b. (x² -1)
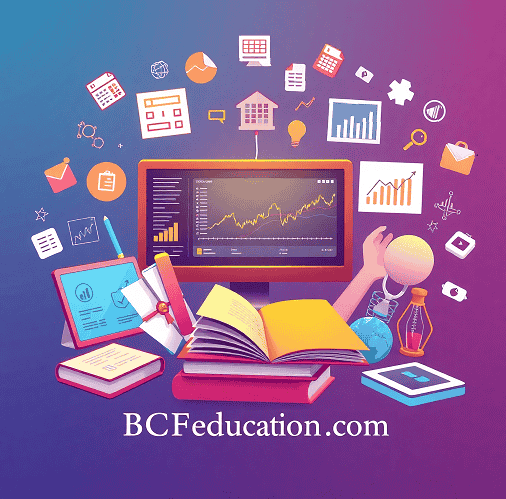
Solution:
X | f(x) | Y = 2x – 3 | Y = x² -1 |
-1 | 0.125 | 2(-1) – 3 = -5 | -1² – 1 = 0 |
0 | 0.50 | 2(0) – 3 = -3 | 0² – 1 = -1 |
1 | 0.20 | 2(1) – 3 = -1 | 1² – 1 = 0 |
2 | 0.05 | 2(2) – 3 = 1 | 2² – 1 = 3 |
3 | 0.125 | 2(3) – 3 = 3 | 3² – 1 = 8 |
(vii) What is a binomial distribution? Write its four important properties.
Answer: Binomial distribution is a function which is used to calculate the binomial experiment.
Function is given below:
for x = 0, 1, 2, 3 …..n.
Where n = sample size, x = number of successes, n – x = number of failure
P =success and q = failure = 1 – p
Properties
- The mean of binomial distribution µ = np
- Variance 𝜎² = npq
- Standard Deviation σ= √npq
- ∑px = 1
- If p = q = ½ then probability distribution is symmetrical
- For negative skewness P>q
- For positive skewness P<q
(viii) A random variable X is believed to follow a binomial distribution b (x;5, p). If P(X=0) =243/1024, find P(X=3).
Solution:
Here n = 5 p = ?, q = ?, P(X = 3) = ?
(ix) Given X is a binomial random variable with parameters n=1 and p. Find its Mean and S.D.
Solution:
(x) What is a Normal distribution? Describe its four properties.
Answer:
Normal Distribution
Normal distribution also called Gaussian Distribution is an ideal arrangement of numbers concentrated in the middle of the range which make bell shaped histogram trails symmetrically right and left. Standard normal distribution has mean = 0 and standard deviation = 1. Standard normal distribution is typically represented by Z which is equals to:
Properties of Normal Distribution
- It is perfectly symmetric around the mean µ.
- Mean, median and mode are located at the center of the distribution.
- Distribution has bell shaped curved located mean at the center.
- Follows empirical rule follows (68-95-99.7) which means:
Approximately 68% of the data falls within (μ±σ) of the mean.
Approximately 95% of the data falls (μ±2σ) of the mean.
Approximately 99.7% of the data falls (μ±3σ) of the mean.
- It is defined by the two parameters μ & σ.
- Normal distribution has single peak value which is at the center of the distribution.
(xi) The standard deviation of a normal distribution is 4. Find its first four moments about mean.
Solution:
Data
S.D σ = 4, Variance σ² = 4²=16
After putting the data:
(xii) Differentiate between Parameter and Statistic. Why is a parameter said to be constant and statistic a variable?
Answer:
Statistical estimator of population is called parameter such as mean and variance of population is parameter whereas statistical estimator of sample is called statistic such as mean and variance of sample is statistic.
Population parameters are considered as constant because they apply on whole population so there is no variation whereas sample statistic is considered as variable because it applies on different samples and results change when sample changes.
(xiii) Given N1 = 3 and N 2 = 3, P1 = ½ and P2 = 1/3, n1 = 2 and n2 = 2. Find E (P̂1 – P̂2) and S.E (P̂1 – P̂2)
Solution:
Here
(xiv) What is an Unbiased estimator? Is it possible to have more than one unbiased estimator for an unknown parameter? Give an example.
Answer:
Estimator is sample statistic which estimates the unknown population parameter. Statistical estimator is unbiased if it is equal to population parameter and is biased when it is not equal to population parameter.
And yes it is possible that there are more than one unbiased estimators for unknown parameter such as:
(xv) A random sample of 400 members of labour force in a certain region showed that 32 were employed. Construct 95% confidence interval for proportion of unemployed in the region.
Solution:
Data: n = 400, employed people = 32, x = unemployed people = 400 – 32 = 368
Test Statistic
Conclusion
0.8934 < p < 0.9465
Proportion of unemployed people in the region is approximately 89.34% to 94.65%
(xvi) Describe the procedure for testing hypothesis about mean of a normal population when population Standard Deviation is unknown and the sample size is small.
Answer:
Sometimes the hypothesis about the population which is normal and its standard deviation 𝜎 is unknown. In this case t test is used both for small sample size. Thus t test is given below:
where X̅ = Sample mean, µ = Population mean, s = sample standard deviation and n = sample size
Following steps will be taken:
Step 1: Stating Hypothesis
Step 2: Level of significance
Step 3: Test Statistic
Step 4: Critical Region
Step 5: Calculation
Step 6: Conclusion
(xvii) A random sample of size 100 is taken from normal population with an S.D of 40. If the sample mean=220, test the hypothesis that μ ≤ 200 at α = 5% level of significance.
Solution:
Data: n = 100, s = 40, x̅ = 220, μ = 200, α = 5%
Step 1 Hypothesis
Ho: µ ≤ 200
H1: µ > 200
Step 2 Level of Significance
α = 0.05
Step 3 Test Statistic
Step 4 Critical Region
Critical Value Z > 1.645
Step 5 Calculation of Z Statistic
Step 6 Conclusion
Calculated Z value falls in rejection region or greater than tabulated value so we reject Ho.
(xviii) If there are 144 A’s and 384 B’s in 1024 observations, how many AB’s and αβ’s are required so that A and B are independent.
Solution:
(A) = 144, (B) = 384, n = 1024
There are 54 AB’s and 550 αβ’s is required so that A and B are independent.
(xix) Write the name of the main computer units.
Answer:
The main computer units are:
- Central Processing Unit (CPU): The brain of the computer responsible for executing instructions and processing data.
- Memory (RAM): Stores temporary data and program instructions that the CPU needs while performing tasks.
- Storage: Long-term data storage, typically provided by hard drives (HDD) or solid-state drives (SSD).
- Input/Output (I/O) Devices: Hardware like keyboards, mice, monitors, and printers used to interact with the computer.
Extensive Questions
Q. 3 (a) One bag contains 4 white balls and 3 black balls. A second bag contains 3 white balls and 5 black balls. One ball is drawn from the first bag and placed unseen in the second bag. What is the probability that a ball now drawn from the second bag is black?
Solution:
Probabilities from the 1st bag
Condition 1:
It is assumed first that ball drawn from first bag is white and when dropped in to second bag then second bag contains 4 red and 7 white balls.
Data: White balls = 3+1=4, Black balls = 5, N = 4 + 5 = 9, Draw = 1
Condition 2:
It is assumed that ball drawn from first bag is black and when dropped in to second bag then second bag contains 3 white and 5+1 = 6 black balls.
Data: White balls = 3, Black balls = 6, N = 9, Draw = 1
(b) Five balls are drawn from a box containing 7 red and 4blue balls. If X denotes the number of red balls drawn from the box, obtain the probability distribution of X. Compute the mean and variance of this distribution. Compare your results with the theory.
Solution:
Data
Red Balls = 7, Blue Balls = 4, Total Balls N=11, Draw (r)=5, X = 1, 2, 3, 4, 5 where 0 is not possible because there are only 4 blue balls and draw is 5 balls
Events
Calculation of Probabilities
X | P(X) | xp(x) | x²p(x) |
1 | 7/462 | 7/462 | 7/462 |
2 | 84/462 | 168/462 | 336/462 |
3 | 210/462 | 630/462 | 1890/462 |
4 | 140/462 | 560/462 | 2240/462 |
5 | 21/462 | 105/462 | 525/462 |
∑p(x) = 462/462 = 1 | ∑xp(x) =1470/462 | ∑x²p(x) =4995/462 |
Q. 4 (a) In a normal distribution Mean is 300 and variance is 100, find: (i) The area above 314 (ii) D8
Solution:
Data
To find the area above 314, we have to calculate:
From the z-table, the area to the left of Z=1.4 is approximately:
P(Z<1.4) = 0.9192
The area above Z=1.4 is:
P(Z>1.4) =1−0.9192=0.0808
Thus, the area above X=314 is: 8.08%
The 8th decile corresponds to the value D8, below which 80% of the data lies. For a normal distribution, this is equivalent to finding the z-score for the cumulative probability P(Z<z)=0.8.
From the z-table, the z-score for P(Z<0.8) is approximately:
Z=0.84
We now convert this z-score back to the corresponding value in the original distribution using the formula:
X=Zσ+μ
Substitute Z=0.84, σ=10, and μ=300:
X=0.84⋅10+300
X =8.4+300
X =308.4
Final Results
- Area above X=314 is: 8.08%
- D8 = 308.4
b. Let X1 represent the means of the sample of size n1 = 2 drawn without replacement from a population consisting of 4,6, 8, 10. Similarly let X2 represent the means of the samples of size n2 = 2 drawn without replacement from another population consisting of 2, 4,6: (i) Find all possible differences between sample means i.e (X̅1 – X̅2). (ii) Construct the sampling distribution of (X̅1 – X̅2) and compute its mean and variance. (iii) Verify the results using formulae.
Solution:
Distribution 1 Calculation
Data
Case: Without Replacement
All possible Samples, their sums and means
Samples | Sum of Samples | Mean of Samples X̅1 |
4, 6 | 10 | 5 |
4, 8 | 12 | 6 |
4, 10 | 14 | 7 |
6, 8 | 14 | 7 |
6, 10 | 16 | 8 |
8, 10 | 18 | 9 |
Distribution 2 Calculation
Data
Case: Without Replacement
All possible Samples, their sums and means
Samples | Sum of Samples | Mean of Samples X̅2 |
2, 4 | 6 | 3 |
2, 6 | 8 | 4 |
4, 6 | 10 | 5 |
Calculation of Difference between means of samples
X̅2 | X̅1 | |||||
5 | 6 | 7 | 7 | 8 | 9 | |
3 | 2 | 3 | 4 | 4 | 5 | 6 |
4 | 1 | 2 | 3 | 3 | 4 | 5 |
5 | 0 | 1 | 2 | 2 | 3 | 4 |
Frequency Distribution of Difference of Sample Means
(d= X̅1 – X̅2) | f | f(d) | df(d) | d²f(d) |
0 | 1 | 1/18 | 0 | 0 |
1 | 2 | 2/18 | 2/18 | 2/18 |
2 | 4 | 4/18 | 8/18 | 16/18 |
3 | 4 | 4/18 | 12/18 | 36/18 |
4 | 4 | 4/18 | 16/18 | 64/18 |
5 | 2 | 2/18 | 10/18 | 50/18 |
6 | 1 | 1/18 | 6/18 | 36/18 |
∑f = 18 | ∑ df(d) = 54/18 | ∑ d²f(d) = 204/18 |
Calculation of Population Parameters of Distribution 1
X1 | 4 | 6 | 8 | 10 | ∑X1 = 28 |
X1² | 16 | 36 | 64 | 100 | ∑X1² = 216 |
Calculation of Population Parameters of Distribution 2
X2 | 2 | 4 | 6 | ∑X2 = 12 | |
X2² | 4 | 16 | 36 | ∑X2² = 56 |
Verification
Q. 5 (a) Samples of sizes n1 =10 and n2 = 15 are drawn from the normal populations with the same but unknown standard deviation. The means of the samples are. X̅1 = 20, X̅2 = 15 and the unbiased sample variances are 16 and 14. Is the difference between sample means significant? Use α= 5%.
Solution:
Data:
Step 1: Hypothesis
Null Hypothesis Ho: μ1=μ2
Alternative Hypothesis : μ1≠μ2
Step 2: Level of Significance α = 0.05
Step 3: Test Statistic
Step 4: Critical Region:
Step 5: Computations
Step 6: Conclusion
Our calculated value of t = 3.18 falls in rejection region so we will reject Null Hypothesis and accept Alternative Hypothesis.
(b) Given the following data: Do the data suggest association between education and gender? Use α = 1%
Education | Middle | High | College |
Gender | |||
Male | 220 | 270 | 110 |
Female | 120 | 45 | 35 |
Solution:
Education | Middle | High | College | Total |
Gender | ||||
Male | 220 | 270 | 110 | 600 |
Female | 120 | 45 | 35 | 200 |
Total | 340 | 315 | 145 | 800 |
Step 1 Testing the Hypothesis
Ho: There is no Association between Level of Education & Gender.
H1: There is Association between Level of Education & Gender.
Step 2 Level of Significance
Level of Significance = α=0.01
Step 3 Test Statistic
Step 4 Critical Region
Critical Region: Degree of Freedom d.f= (R-1)(C-1)
So d.f= (2-1)(3-1)=2
The Value of Tabulated χ²(0.01,2)=9.210
The Critical Region χ²cal>9.210
Step 5 Calculation of Expected Frequencies
Step 6 Calculation of χ²:
Table Computation of χ² | ||||
fo | fe | fo-fe | (fo-fe)² | |
220 | 255 | -35 | 1225 | 4.804 |
270 | 236.25 | 33.75 | 1139.063 | 4.821 |
110 | 108.75 | 1.25 | 1.5625 | 0.014 |
120 | 85 | 35 | 1225 | 14.412 |
45 | 78.75 | -33.75 | 1139.063 | 14.464 |
35 | 36.25 | -1.25 | 1.5625 | 0.043 |
38.559 | ||||
|
Step 7 Conclusion
The calculated value of χ² is 38.559 is more than the tabulated value of χ² 9.210 or 38.559 falls in the rejection region. We reject the Null Hypothesis Ho, accepts alternative hypothesis H1 and conclude that there is association between Level of Education & Gender.
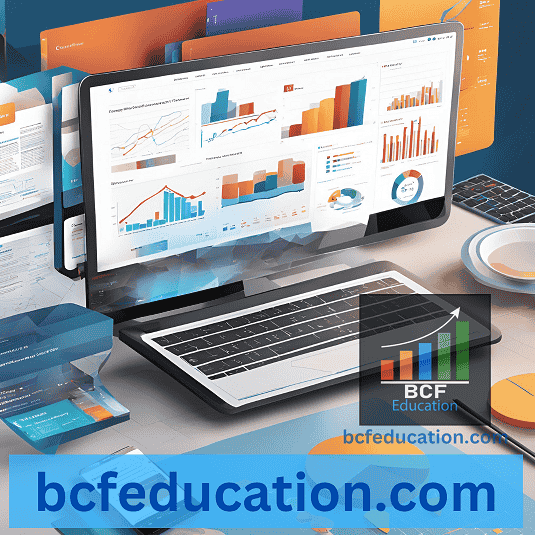
Related Articles